

COVID-19 causes a spectrum of symptoms ranging from mild to severe pneumonia as well as asymptomatic cases. As of Febru(based on the WHO statistics), over 430 million confirmed cases and over 5.7 million deaths have been recorded (2022). IntroductionĬoronavirus disease 2019 (COVID-19) is a newly recognized illness, caused by the highly contagious severe acute respiratory syndrome coronavirus 2 (SARS-CoV-2) and spread rapidly around the world in the last two years ( Hong et al., 2021). Interleukin-10 is a useful predictor of critically ill patients with COVID-19 pneumonia.

The nomogram and visualized interpretation with LIME plot could be useful in the clinical setting.ģ. XGBoost exhibited the highest discriminatory performance for prediction of critically ill patients with COVID-19 pneumonia.Ģ. Additionally, interleukin-10 could serve as a useful predictor of critically ill patients with COVID-19 pneumonia.ġ. It is inferred that the nomogram and visualized interpretation with LIME plot could be useful in the clinical setting. Individualized prediction by XGBoost model was explained by local interpretable model-agnostic explanations (LIME) plot.Ĭonclusions: XGBoost exhibited the highest discriminatory performance for prediction of critically ill patients with COVID-19 pneumonia. The mean AUC for LR, RF, and XGBoost model were 0.91, 0.89, and 0.93 respectively (in two-fold cross-validation). RF and XGBoost methods suggested that Interleukin-10 and interleukin-6 were the most important variables for severity of illness prediction. The coefficients from LR model were utilized to build a nomogram. Ten variables (respiratory rate, neutrophil counts, aspartate transaminase, albumin, serum procalcitonin, D-dimer and B-type natriuretic peptide, CD4 + T cells, interleukin-6 and interleukin-10) were used as candidate predictors for LR model, Random Forest (RF) and XGBoost model application. Interleukin-10 with an AUC of 0.86 was most useful predictor of critically ill patients with COVID-19 pneumonia. Results: Univariate analysis revealed that there was a difference between critically and non-critically ill patients with respect to levels of interleukin-6, interleukin-10, T cells, CD4 + T, and CD8 + T cells. The performance of these models was measured by area under receiver operating characteristic curve (AUC) analysis. A logistic regression function (LR model), Random Forest, and XGBoost models were developed. Outcomes were followed up until Mar 12, 2020. Methods: Clinical symptoms and signs, laboratory parameters, cytokine profile, and immune cellular data of 63 COVID-19 pneumonia patients were retrospectively reviewed. 9Department of Intensive Care Unit, The First Affiliated Hospital of Wenzhou Medical University, Wenzhou, Chinaīackground and Aims: The aim of this study was to apply machine learning models and a nomogram to differentiate critically ill from non-critically ill COVID-19 pneumonia patients.8Unit of General Surgery, Sandro Pertini Hospital, Rome, Italy.7Department of Surgery, World Mate Emergency Hospital, Battambang, Cambodia.6Department of Gastroenterology, Scientific Research Institute of Medical Problems of the North, Krasnoyarsk, Russia.5Internal Medicine Unit, Budrio Hospital, Bologna, Italy.4Unit of Gastroenterology and Digestive Endoscopy, Sandro Pertini Hospital, Rome, Italy.Panjwani Centre for Molecular Medicine and Drug Research, International Center for Chemical and Biological Sciences, University of Karachi, Karachi, Pakistan 3Jamil-ur-Rahman Center for Genome Research, Dr.
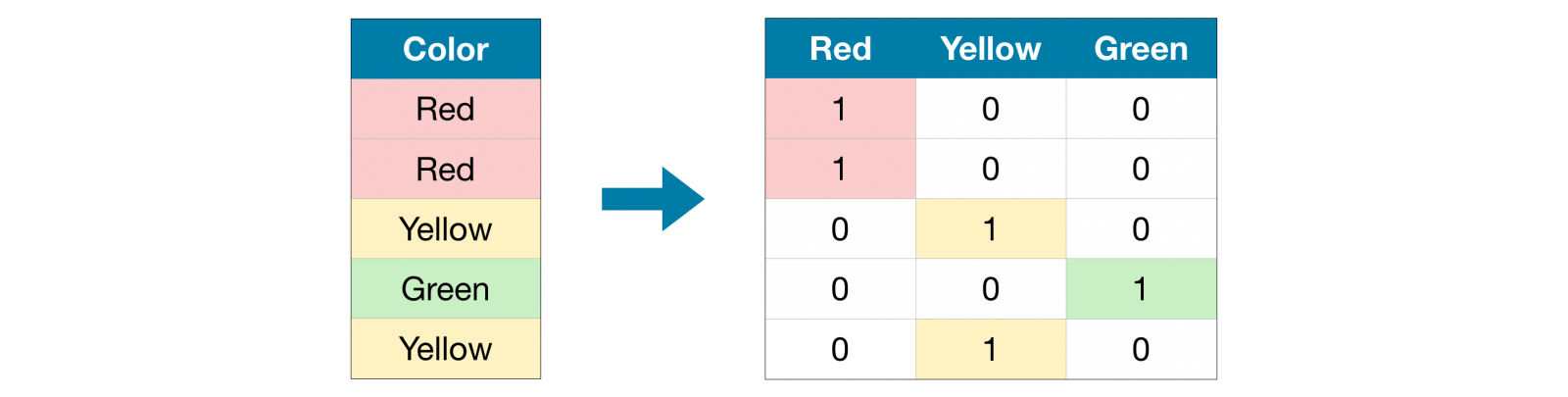
2School of the First Clinical Medical Sciences, Wenzhou Medical University, Wenzhou, China.1Department of Gastroenterology and Hepatology, The First Affiliated Hospital of Wenzhou Medical University, Wenzhou, China.Wandong Hong 1*† Xiaoying Zhou 2 Shengchun Jin 2 Yajing Lu 2 Jingyi Pan 2 Qingyi Lin 2 Shaopeng Yang 2 Tingting Xu 2 Zarrin Basharat 3† Maddalena Zippi 4 Sirio Fiorino 5 Vladislav Tsukanov 6 Simon Stock 7 Alfonso Grottesi 8 Qin Chen 9 Jingye Pan 9*
